- Published on
HICRIC: Law, Policy, and Medical Guidance for Health Insurance Coverage Understanding
- Authors
- Name
- Mike Gartner, PhD
This week we released two datasets we've been developing for a long time.
These datasets provide a foundation for those (like us!) developing machine learning applications to support patients fighting inappropriate coverage denials.
The Health Insurance Coverage Rules Interpretation Corpus (HICRIC) is a curated collection of reputable legal and medical text designed to support applications that require understanding of U.S. health insurance coverage rules. We use this data internally to train models that automatically generate high quality appeal letters for patients facing inappropriate denials.
Our external appeal adjudication benchmark was designed to support prediction of external appeal outcomes. The goal is to help regulators lower mean time to overturn for inappropriate denials, and to help inform patients of their rights, and their case-specific likelihood of accessing care, to increase utilization of appeal recourse. Many patients forgo their appeal rights under the mistaken belief that they are unlikely to win an appeal.
You can read more about the motivation and technical aspects of this work in our forthcoming paper.
HICRIC Corpus
Our dataset consists of documents from diverse sources. It includes U.S. federal and state law, insurance contracts, official regulatory guidance, agency opinions and policy briefs, official coverage rules for Medicaid and Medicare, and summaries of appeal adjudications.
Each document in our corpus belongs to exactly one of the following categories:
Legal.
Current or former U.S. law.
Regulatory Guidance.
Guidance on U.S. law, released by agencies.
Coverage Rules, Contracts, and Medical Policies.
Text outside formal law that describes binding coverage rules. This includes text from contracts, and contract-referenced or proprietary medical policies.
Opinion, Policy, and Summary.
Opinions, policy perspectives, or summaries of law, proposed law, executive actions, or compliance.
Case Descriptions.
Reviews of individual health insurance coverage decisions.
Clinical Guidelines and Medical Literature.
Clinical guidelines and medical literature, excluding contract-specific medical policy falling into category 3.
In total, the dataset contains 8,311 documents, 419 million words, and 2.7 billion characters.
A high level characterization of the distribution of text in our corpus in terms of this breakdown is below:
Category | Num Documents | Words | Chars | Size (GB) |
---|---|---|---|---|
All | 8,310 | 417,617,646 | 2,699,256,987 | 2.81 |
legal | 335 | 92,357,802 | 596,044,008 | 0.60 |
regulatory-guidance | 1,110 | 5,536,585 | 38,607,587 | 0.04 |
contract-coverage-rule-medical-policy | 7 | 196,156,813 | 1,228,184,524 | 1.31 |
opinion-policy-summary | 2,094 | 19,462,399 | 133,049,956 | 0.14 |
case-description | 2,629 | 214,267,074 | 1,351,074,791 | 1.45 |
clinical-guidelines | 2,150 | 81,955,020 | 553,041,990 | 0.56 |
External Appeal Prediction Benchmark
Our external appeal prediction benchmark is designed to support the following task:
External Appeal Adjudication Task Given a description of a denial, predict whether an external appeal would result in overturn, uphold, or whether the description is insufficient to make such a prediction.
Our benchmark consists of (Background Context, External Appeal Outcome, Sufficiency Label) triples.
The background context is brief, non-leaking context extracted from real adjudication summaries. The outcomes are actual binding case determinations made by independent medical reviewers. The sufficiency label is a binary pseudo-label indicating the extent to which the background context is sufficient to make an informed prediction about the expected case outcome.
Here is an example from the benchmark:
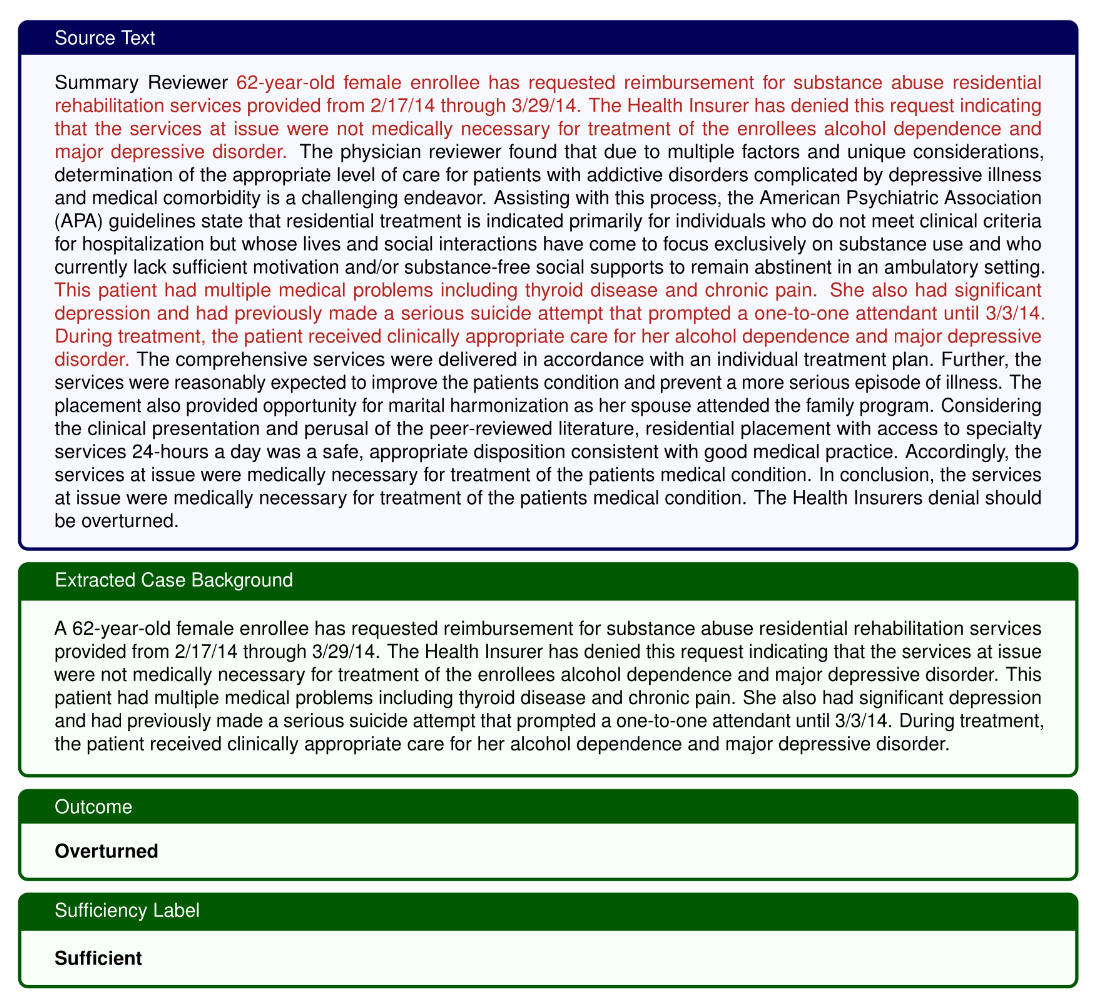
An example from our benchmark. This example is actually a bad one, as the words "clinically appropriate" in the extracted text leak the case outcome, but it hopefully illustrates the goal. We are actively improving our pipeline and performing larger scale annotation to improve issues such as this one. Our benchmark includes many high quality examples, but we thought it would be best to illustrate the subtleties and difficulties via an imperfect example here.
We are using this benchmark to develop our free external appeal overturn prediction models.
We are actively developing this benchmark, and our current release is a V0 prototype. If you'd like to get involved in the ongoing annotation effort, particularly if you are a doctor, nurse, or attorney, please get in touch!
Access the Data
If you are interested in using this data to support patients and protect their rights, you can get started with the data right away.
We are releasing this data to all for free, under a permissive license, in the hopes that it spurs ML development towards these ends. Unlike many venture funded efforts in this space, our primary goal is systemic change for the benefit of patients, and we are willing to prioritize that over our own organizational success.
Support Our work
That said, our biased opinion is that the free support and tools we provide to patients contributes a lot of value to improving U.S. healthcare. We've helped many patients access care critical to their wellbeing, and have gotten over $300k in inappropriate denials overturned on behalf of patients. While our services are free, we are actively seeking funding to make our work sustainable, if you are looking for a cause to donate towards 😂 Your support allows us to remain focused on systemic change, not enriching shareholders.
Get Involved
If you find the data useful, or are interested in getting involved in our efforts, we'd love to hear from you. Please feel free to reach out with questions, comments, or feedback to info@persius.org.