- Published on
Toward Generative Contract Understanding Tools
- Authors
- Name
- Mike Gartner, PhD
Introduction
Health insurance claims denials in the U.S. pose serious problems for patients. They cause delays, forgone care, and detrimental health outcomes. Sometimes patients receive services despite denials, incurring bills, debt, and collections lawsuits. For the most vulnerable, there are calamitous effects (Pollitz et al., 2023), (Collins et al., 2023), (Lopes et al., 2022).
Evaluating claims necessitates precise understanding of a complex web of case-specific laws, contracts, and medical literature. Specialists such as medical coders, pharmacists, doctors, and attorneys perform the work, which is manual, time consuming, and rate-limited by research.
Advances in natural language understanding (NLU) present an opportunity to support case workers and improve patient outcomes. In particular, generative question answering (QA) models can increase the efficiency with which specialists understand cases. However, there are barriers preventing responsible and effective use of such tools.
Background
Claims denials are valuable and far-reaching. Conservatively, hundreds of millions of claims worth billions of dollars are denied each year (Pollitz, Lo, et al., 2023) (Gartner, 2023). One estimate suggests 30% of Americans have healthcare debt related to bills they expected insurance to cover (Lopes et al., 2022).
While some denials are warranted, there is a growing body of evidence that suggests inappropriate denials are pervasive, systemic, and inequitably distributed (Levinson, 2018), (Grimm, 2022), (Grimm, 2023), (Armstrong et al., 2023), (Ross & Herman, 2023), (Gartner, 2023). Mechanisms exist to support patients seeking recourse, but they are underutilized and overburdened (Pollitz, Lo, et al., 2023), (Gartner, 2023). Tools that support efficient and accurate contract understanding stand to serve patients and case workers alike.
Barriers to Deployment
Existing language models (e.g. (OpenAI et al., 2023) or (Touvron et al., 2023)) can be deployed to serve as generative support tools. Coupled with inference-time retrieval (Lewis et al., 2020), this approach can be effective (cf. Figure 1).
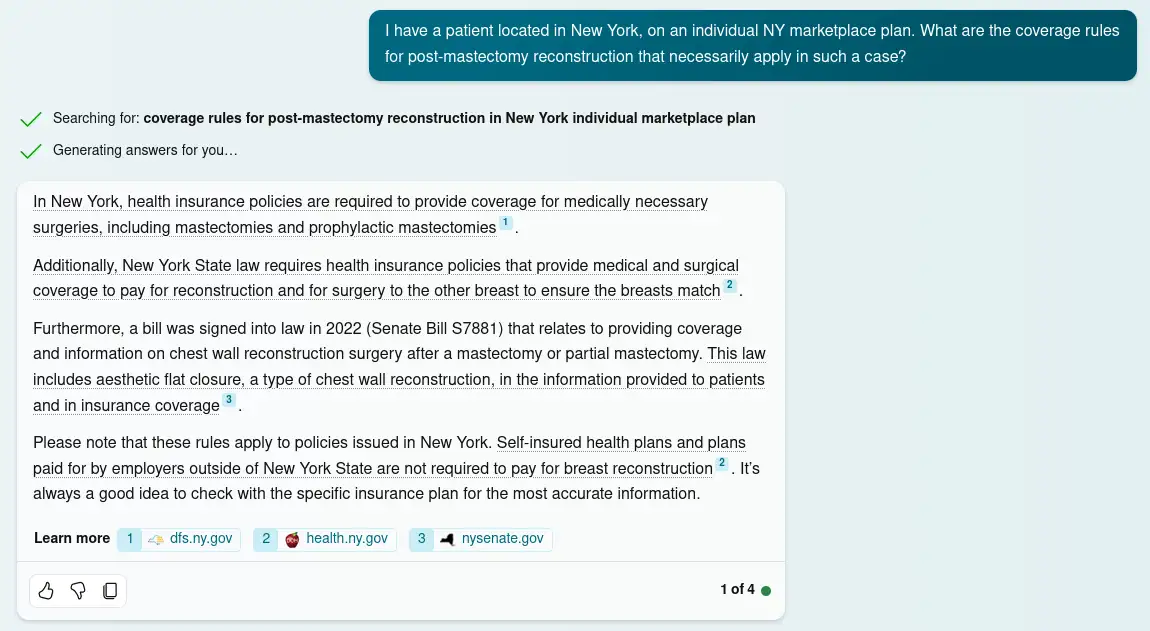
Figure 1.
Bing Chat's response to a question about an Affordable Care Act compliant NY health plan includes relevant, authoritative supporting documentation backing up its claims. The incorporation of information from retrieved documents, and attribution of individual claims to those documents, are desirable features that allow the output to be systematically audited for quality as part of responsible use by a case worker. Date accessed: 1/9/24. Conversation style set to 'precise'.
However, there are at least two barriers to responsibly deploying this approach:
- Hallucinations are common (Ji et al., 2022) (Huang et al., 2023).
- There is a dearth of domain-specific datasets sufficient for use as knowledge bases.
The first barrier diminishes the utility of generative support tools lacking built-in verification mechanisms. Such tools place an additional burden on case workers engaged in responsible use. While correct claims may streamline research, incorrect claims require extra work to debunk. More concerningly, users that forgo verification jeopardize case outcomes. Figure 2 shows a relevant example.
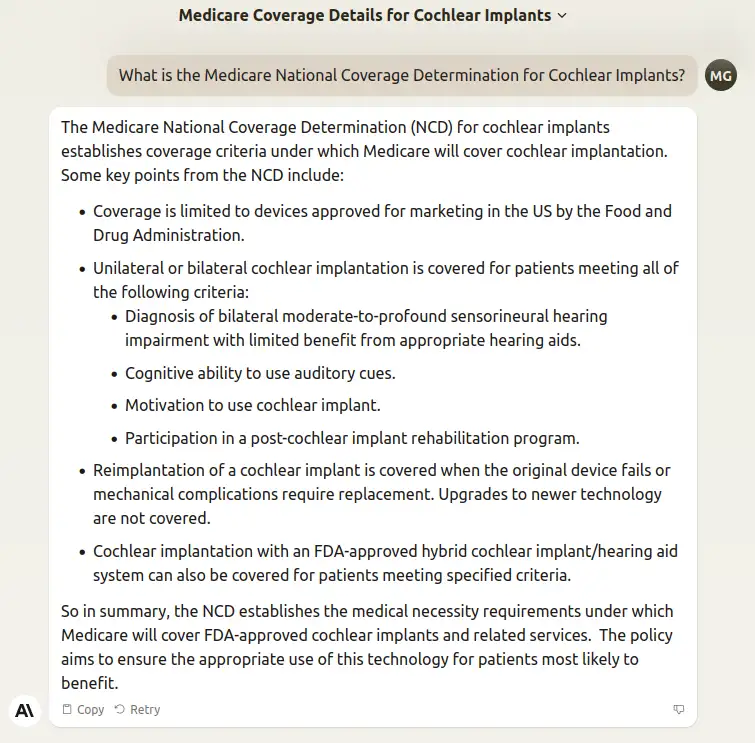
Figure 2.
Anthropic's Claude responds to a question about the Medicare National Coverage Determination for cochlear implants. The response provides an accurate summary of key points, but omits criteria stated in the NCD necessary to determine coverage in individual cases. As a result, applying the unverified response to the facts of a case has a nontrivial likelihood of resulting in an incorrect conclusion. Date accessed: 1/9/24.
Retrieval-supplemented language models rely on their indexed corpora. Lack of a domain-specific dataset often leads existing models to inappropriately rely on non-authoritative documents, or lack requisite knowledge, as seen in Figure 3.
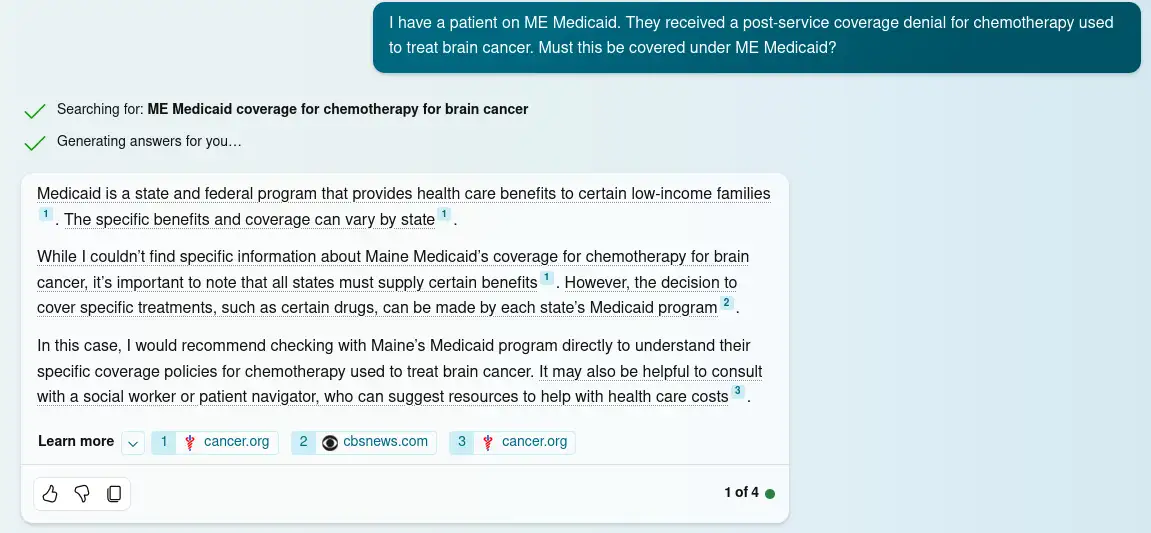
Figure 3.
Bing Chat's response to a question about coverage rules in Maine's Medicaid program, MaineCare. The chat pipeline's knowledge base index apparently either lacks access to relevant authoritative sources (such as the the MaineCare Member Handbook) that can help address the question, or alternatively the model may simply be erring in failing to retrieve them in this case. The response is too generic to provide any utility to a skilled caseworker. Date accessed: 1/9/24. Conversation style set to 'precise'.
These deficiencies, coupled with the dire implications of negative case outcomes for patients, make systematic utilization of existing systems modestly effective at best, and precarious and fraught at worst.
Addressing Deficiencies
Addressing hallucinations is a highly active field of research (Towhidul Islam Tonmoy et al., 2024). Use of Retrieval Augmented Generation (Lewis et al., 2020) produces systematically verifiable outputs, which we hypothesize would sufficiently mitigate hallucinations when used with the right knowledge base. Models tailored to span-selection based QA (Rajpurkar et al., 2018), (Ram et al., 2021) are compelling in that they forgo the hallucination problem altogether. Approaches to such QA that incorporate language model representations suggest a promising, alternative approach.
There is a pressing need for a comprehensive dataset of authoritative documents to enable fine-tuning and knowledge base construction. While high-quality pre-training data exists in the public domain, much of it cannot be legally scraped. Other key data is never publicly disseminated. High-quality corpora have contributed to the rise of many successful legal NLU applications (Elwany et al., 2019) (Hendrycks et al., 2021) (Wang et al., 2023) (Koreeda & Manning, 2021), and we expect the same will be true in this domain. We are actively curating such a dataset now, and hope to release it and use it to train prototype case support tools in the near future.
References